Data scientists know more than anyone else that good quality data is the foundation for useful artificial intelligence (AI) and machine learning (ML) models. Only when data quality is good, usable and from multiple sources is when the real potential of AI and ML can be leveraged.
Right now, data scientists and analysts spend a ridiculous amount of time and money preparing the data. Too often this includes anonymization techniques that protect personally identifiable information (PII) but render the data useless or dumb. Additionally, current solutions don’t provide a method to combine and match anonymized individuals from multiple data sources. After all, how can you join anonymous data?
This is how it has always been but is that still true? What if data scientists and analysts could link anonymous data, from any number of sources, and get all the value out of it as if it were identified and with no risk?
Poly-Anonymization: Stop Using PII, Keep Data Smart and Get Results
Anonymization and privacy protection techniques like differential privacy and tokenization are still being used even though it’s common knowledge they are neither secure nor accurate. They are still being used because they are considered the only data anonymization tools available. This was the challenge Anonomatic faced when asked to help The L.A. Trust for Children’s Health gain insights to better understand the relationship between student health and academic success. To do this, The L.A. Trust needed to collect, combine, and receive vital data from multiple healthcare providers like Planned Parenthood who adhere to HIPAA privacy laws and educators who must follow FERPA compliance. It was a big project; a huge challenge and it quickly became obvious there was no solution available which provided all the capabilities they needed. The conclusion was we needed a new data anonymization and privacy compliance approach, which is how we came up with Poly-Anonymization™ – a key component in our PII Vault ™ solution.
Poly-Anonymization involves taking any personal identifying pieces of information (name, gender, address, social security number, etc.) and swapping it out for our Poly-Anonymous Identifier (Poly-Id). Poly-Id values are unique, inconsistent, unpredictable, have multiple potential values and are not hashed. After data has been poly-anonymized, data scientists, analysts and researchers can easily share the resulting data either internally or externally without the usual risk of loss and exposure of PII. They can now combine any number of poly-anonymized data sets, at the individual level, without every receiving any PII. This makes robust and smart insights, AL and ML models more attainable.
Poly-anonymization helped create the L.A. Trust Data xChange. This data analytics platform relies on PII Vault to join confidential and anonymized student health care academic data to advance wellness and success.
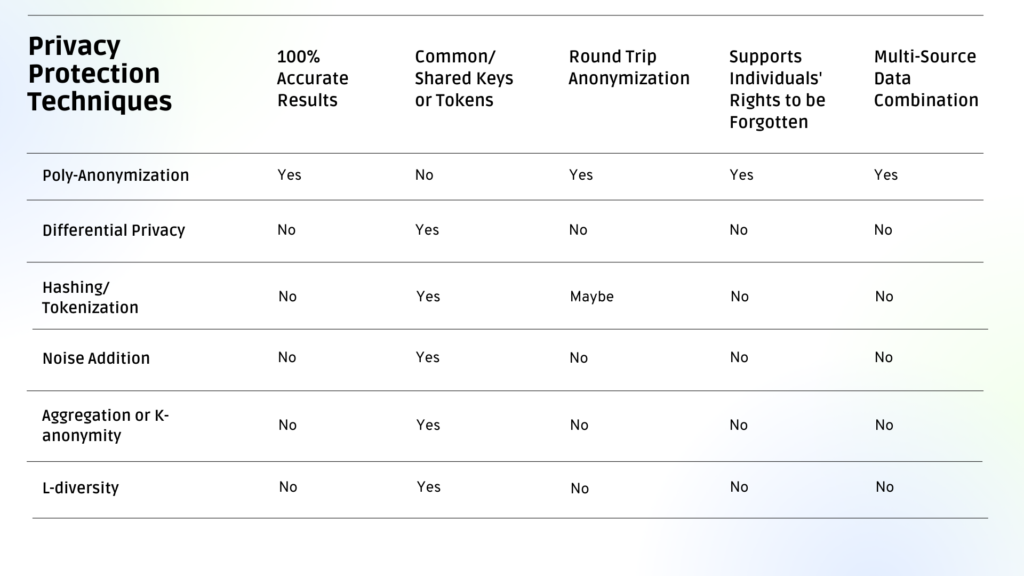
Anonymizing Identified Data and Having that Data Usable Should be Simple
PII Vault addresses major privacy compliance challenges. We know data scientists and analysts don’t want to collect PII but feel they must in order to link data at the individual level. PII Vault addresses this challenge by separating PII from non-PII or fact data and attaching Poly-Ids to the now anonymous fact data. Separating PII data into a completely different environment reduces risks immediately.
This eliminates the need to collect PII data. Every data record received is stamped with an anonymous ID. PII Vault makes it known which anonymous IDs belong to the same person. When data scientists, analysts and researchers don’t need to touch PII, everything is faster, cheaper, and easier as well as 100% accurate. It really can be that simple.
Additional Resources for Data Scientists to Learn More about Anonomatic PII Vault and Poly-Anonymization
We recently added a new capability to PII Vault called Pass-Through Anonymization, which transforms any identified data into safe, anonymous data, that can yield all the value of identified data in a single, simple step. For additional details, check out our white paper titled “A Simple Way to Reduce Risks and Costs Associated with Handling PII Data”. We also just released a white paper on GDPR international data sharing requirements titled “Complying with European Data Protection Board Supplementary Measures”. And we encourage you to start a free trial of PII Vault, where you can test our API-based product on our public cloud using your test data.
Article originally appeared in Data Science Salon Roundtable